Why Slow Thinking Matters for AI
AI excels at fast processing, but is slow thinking the missing piece? This post explores why deliberate reasoning is crucial for developing truly intelligent machines.
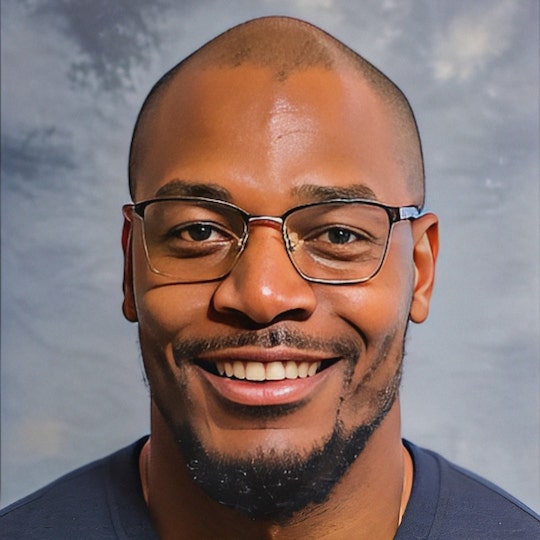
6 min read
Fast and Slow Thinking
Created using Dall•E-3
In the insightful book “Thinking, Fast and Slow,” Daniel Kahneman investigates the ideas of the quick-thinking and slow-thinking brain, known as System 1 and System 2. These represent the different ways we make decisions in our minds.
System 1 (Fast Thinking)
This part of our brain works without us being aware. It’s automatic and doesn’t need any effort. It’s fast, dealing with our immediate and emotional responses. System 1 is like the brain’s automatic pilot, handling most of our thinking. For example, if someone asks you about the existence of a pink elephant, you’d quickly reply based on what you already know. It’s great at handling tasks we’re familiar with, making them into habits, and quickly sorting through information to focus on what’s important, often using shortcuts, called heuristics.
System 2 (Slow Thinking)
This is the opposite of System 1. System 2 requires our attention and conscious effort. It’s where our controlled thinking and logical analysis happen. We use it for careful decisions and when we need to find new or specific information. It doesn’t work as much as System 1, but it’s important, especially when System 1’s quick suggestions might not be enough. It’s a bit like our brain’s ‘energy saver’ mode because it requires more mental energy and effort. For instance, regarding the pink elephant, System 2 would make you double-check and search online, leading you to discover the rare albino pink elephant in Kruger National Park, South Africa.
Created using Dall•E-3
The ‘Fast Thinking’ in AI
It resembles the automated, speedy decision-making observed in machine learning systems. These systems make rapid decisions based on a set of pre-trained data and answering is similar to the process known as Input-Output Prompting (IO).
‘Slow Thinking’ in AI
Mirrors the more measured, reasoning processes which requires more computing power. AI models or when human guidance is required in decision-making.
To Refine AI’s Thinking
Several strategies are employed to enhance AI, one of which is the ‘Chain of Thought’ method. This approach prompts AI to articulate its reasoning step by step, making its thought process transparent. “Self-consistency” ensures that AI’s responses are coherent and logical. The “Tree of Thought” model encourages AI to explore and present diverse viewpoints or solutions, reflecting a branching thought process. This method resonates with the notion of deliberate thinking, though it has its limitations, which will be elaborated on later.
Just as human quick thinking can be biased and rely on heuristics (mental shortcuts), AI systems can exhibit similar biases, especially if these biases are embedded in the training data. For instance, if an AI system is not trained with any form of data on albino pink elephants, and a user asks about their existence, the system might hastily conclude that they don’t exist, mirroring a bias similar to those in humans who might believe pink elephants are purely fictional.
Understanding these biases and the shortcuts through which they manifest in human cognition offers crucial insights into how we can identify, mitigate, and manage such biases in AI.
Meta’s Self-Rewarding Language Models (SRLMs)
Meta’s Self-Rewarding Language Models (SRLMs) signify a crucial leap forward in AI’s capability for self-development and refined decision-making, indicating a future where models can autonomously perfect their training. This advancement, intertwined with insights from Kahneman’s “Thinking, Fast and Slow,” and the functionalities of platforms like AutoGen and many more similar platforms, is revolutionising AI’s trajectory:
Meta’s Self-Rewarding Language Models:
- Self-Enhancement Mechanism: SRLMs from Meta are engineered to continuously improve their performance by generating, evaluating, and learning from their own responses, establishing a cycle of self-improvement. This feature enables AI to evolve its decision-making skills independently, gradually reducing biases and enhancing precision.
- Mitigating Biases and Hallucinations: The self-review and enhancement feature in SRLMs address common AI issues like biases and hallucinations. By enabling the model to scrutinise and prioritise its decisions, it fine-tunes its knowledge base for future tasks, leading to more credible and accurate outcomes.
- Utilising External Resources: Providing SRLMs with access to external data and tools, such as online sources for fact-checking, further bolsters these models. This allows them to verify and augment the information they generate, ensuring more informed and precise decision-making.
Integration with Kahneman’s Cognitive Theory:
- Fast and Slow Thinking in AI: Linking Meta’s SRLMs to Kahneman’s concepts, the rapid decision-making reflects System 1’s instinctive responses, while the slow, methodical review process embodies System 2’s deliberate thinking. This fusion allows AI to react swiftly when needed and engage in in-depth analysis when necessary.
- Continuous Learning and Adaptation: Echoing the human cognitive process of leveraging both fast and slow thinking for learning and adaptation, SRLM method and tools like AutoGen empower AI systems to constantly evolve and refine their capabilities. This perpetual enhancement helps in reducing errors and aligning AI’s decision-making more closely with human-like reasoning.
Capabilities and Practical Applications of Platforms like AutoGen:
- Complex Multi-Agent Dialogues: AutoGen’s multi-agent setup enables intricate interactions among various AI agents, each representing different elements of fast and slow thinking. This can simulate human-like discussions, resulting in more nuanced and thoughtful outcomes.
- Domain Specialisation and Expertise: AutoGen allows for the creation of specialised agents, promoting deep domain-specific knowledge. This specialisation is akin to human expertise in certain cognitive areas, ensuring high accuracy and quality in responses.
- Balancing Speed and Precision: The emphasis on not just quickness but also accuracy in AI decision-making reflects the balance between System 1 and System 2 thinking. While prompt responses are valuable, ensuring precision, even if it requires more time (akin to slow thinking), is crucial, particularly for complex or high-stakes decisions.
Ready to build something?
Embrace the future of business operations with our comprehensive computer vision platform. Empower your team to annotate training data, train powerful models, and deploy them with ease.